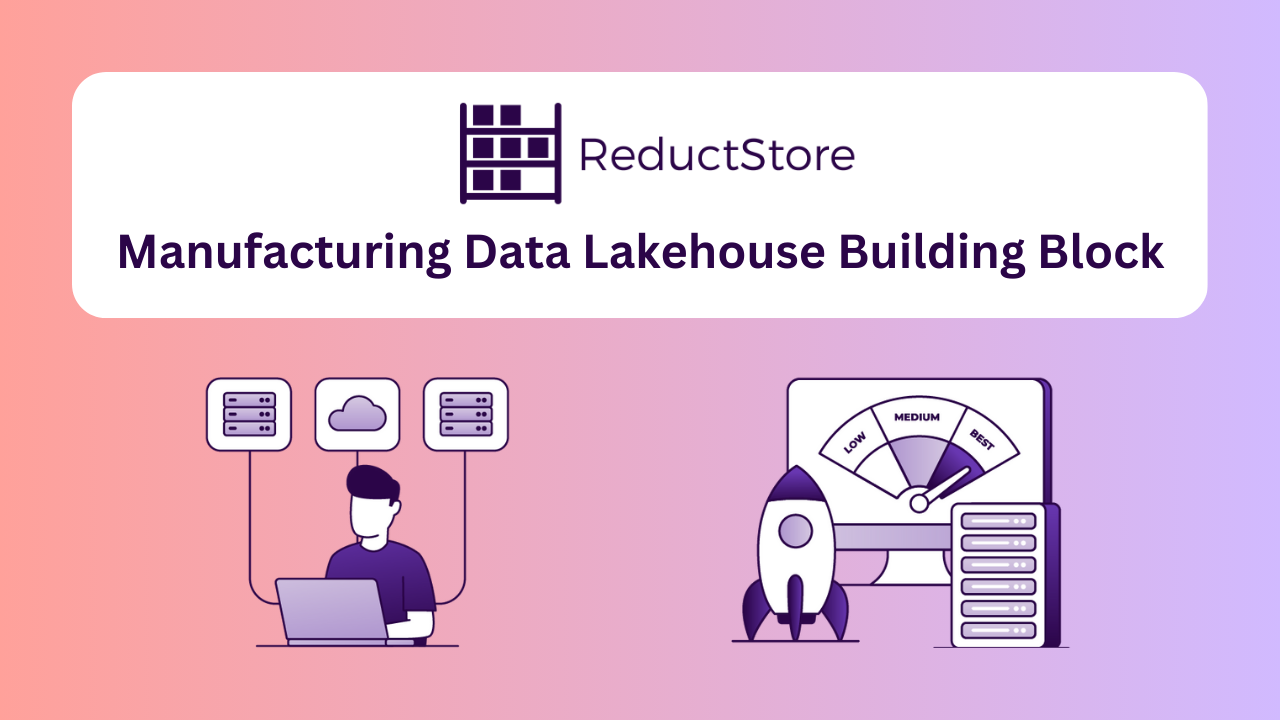
In today's data landscape, flexibility in terms of performance, cost, and storage availability is at a premium. Data warehouses provide structured, analytical capabilities to process large amounts of data. Data lakes, on the other hand, are known for scalability, and the flexibility to handle vast amounts of unstructured data.
In recent years, however, demand has grown for a robust marriage of these two concepts, leveraging cloud-based technologies and advanced data processing frameworks to store massive volumes of data in raw form (data lake), while also supporting structured querying and analytics (data warehouse). This combined data solution is referred to as a data lakehouse.
The data lakehouse concept is particularly useful for manufacturing, as manufacturing requires fast processing of large amounts of data from numerous sources, including sensors (vibration, temperature, power), employee productivity data (files, spreadsheets, documents), logs, cameras, GPS, and more, creating an unstructured jumble of formats that is difficult to process in a traditional data warehouse.
A data lakehouse is an IT infrastructure that provides a unified solution to handle multiple data formats while still providing the capacity to make sense of this data. It supports intelligent query-based analytics and applies structure to the chaos.
At the same time, ReductStore Cloud Solution is a special type of data storage solution that combines the flexibility of a time series database with the capacity of an object storage. In this article, we will explain these strengths in detail and why we think ReductStore has many advantages as a building block to create a data lakehouse for manufacturing.
In order to present these strengths, we will tie our case to the core components of a strong data lakehouse solution.