High Performance Storage and Streaming Solution
An ELT-based solution for robotics and industrial IoT data acquisition systems. Capture data in its raw form, ingest and stream data of any size-images, sensor readings, logs, files, ROS bags-and store it with time indexing and labels for ultra-fast retrieval and management.
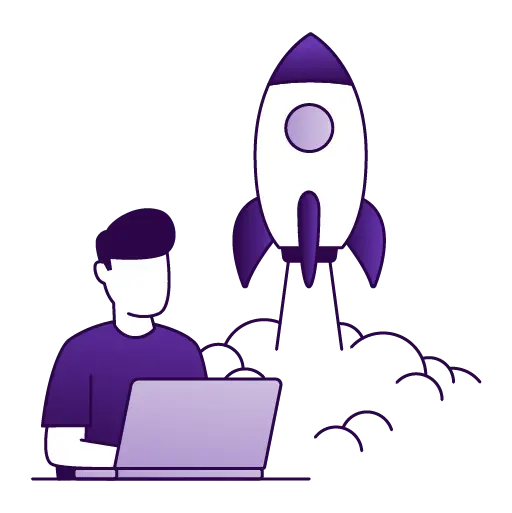
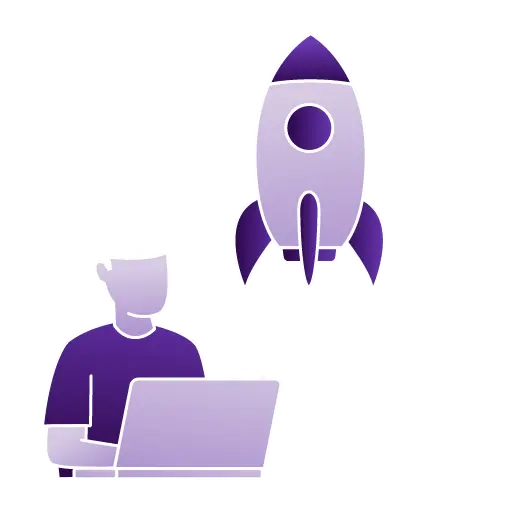
⚡ Ultra-Fast Ingestion & Access
Capture and retrieve raw sensor data, images, LiDAR, logs, and more—10x faster than traditional time series databases. Designed for high-throughput robotics and IIoT workloads.
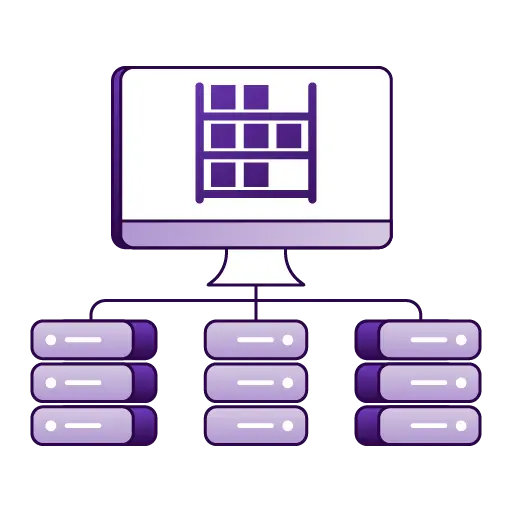
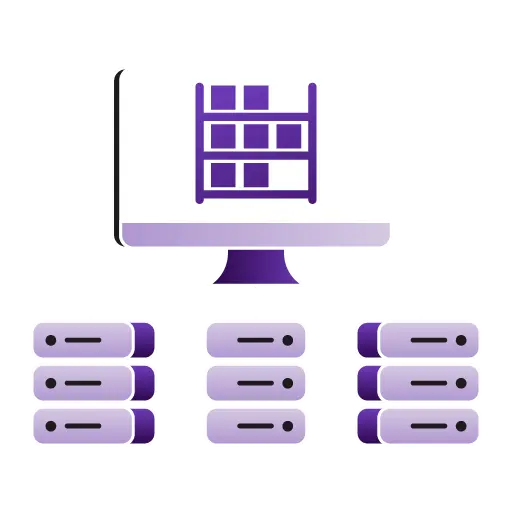
💰 Built for Scale, Not Cost
Store billions of time-indexed records at the edge and in the cloud, and automatically offload cold data to reduce infrastructure costs by up to 90%.
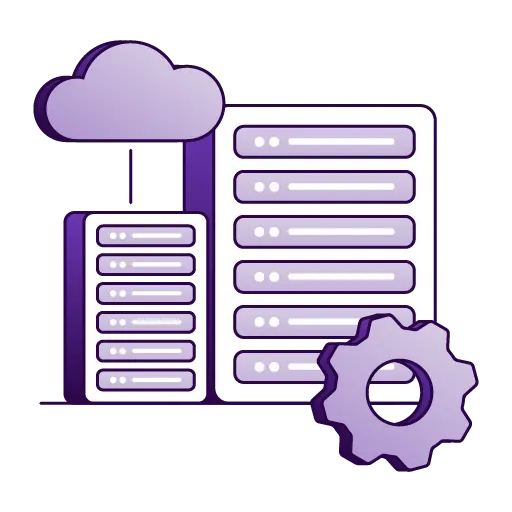
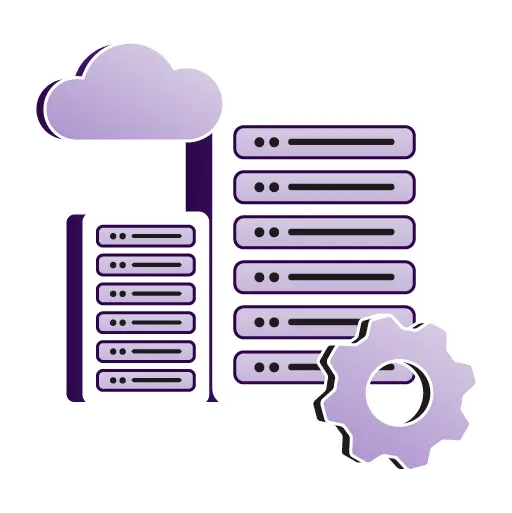
🔒 Reliable by Design - Edge to Cloud
Stream, store, and replicate critical data even with poor connectivity. Avoid data loss, disk overflow, and sync only what matters with label-based filtering and automated retention.
Developers choose ReductStore
Trusted by robotics and IIoT engineers to process billions of time-indexed records 10x faster at 1/10 the cost of traditional storage solutions.
Time Series Blob Storage
Capture and access blob data as time series, tailored for edge computing, computer vision, and IoT.
No Size Limit for Blobs
ReductStore handles blob data without size limits; your disk capacity is the only boundary.
Real-Time FIFO Quota
Ensure optimal storage management with FIFO quotas, preventing disk space shortages in real-time.
Data Labeling & Filtering
Manage your time-series blob data with ease: annotate, filter, and save AI labels or meta-data.
Advanced HTTP(S) API
Integrate and communicate with ReductStore using our feature-rich and secure API.
Efficient Data Batching
Minimize network overhead in areas with high latency by fetching records in batched HTTP responses.
Data Replication
Synchronize data across buckets with replication for high availability and disaster recovery.
Iterative Data Querying
Efficiently queries large datasets with minimal load for real-time and historical data processing.
Token Authorization
Secure data access with token-based authorization to protect your data from unauthorized access.
- Python
- JavaScript
- C++
- Rust
- cURL
import time
import asyncio
from reduct import Client, Bucket
async def main():
client = Client('http://127.0.0.1:8383')
bucket: Bucket = await client.create_bucket("my-bucket", exist_ok=True)
ts = time.time_ns() / 1000
await bucket.write("entry-1", b"Hey!!", ts)
async with bucket.read("entry-1", ts) as record:
data = await record.read_all()
print(data)
loop = asyncio.get_event_loop()
loop.run_until_complete(main())
Client SDKs
You can use the following client SDKs for quick and easy integration into your applications and infrastructure:
Try SDKs →Web Console
ReductStore has an integrated web console that allows you to easily manage your data and access to it.
Try Web Console →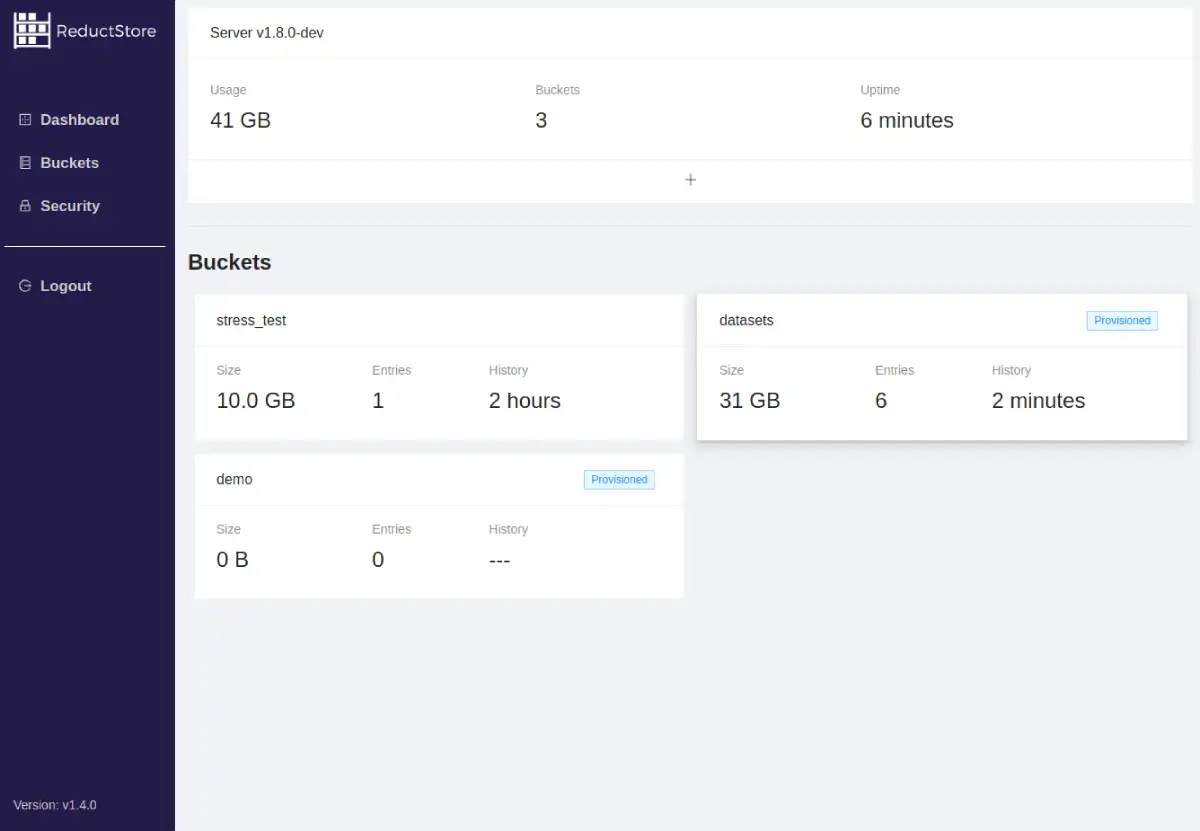
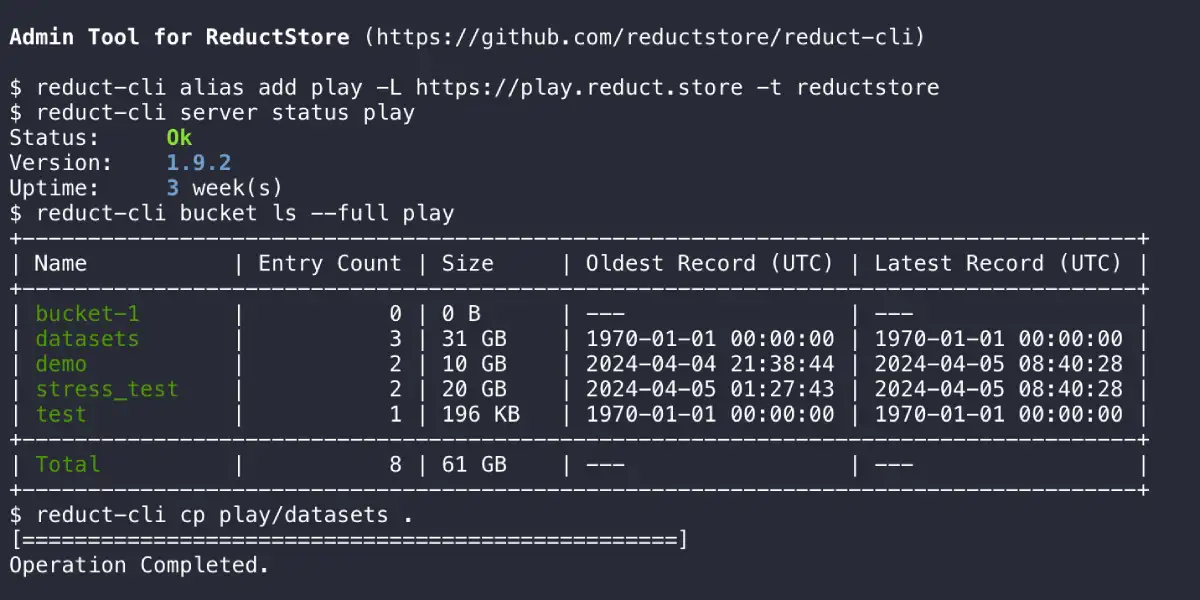
CLI Client
You can customize data retention and replication policies using the ReductStore CLI client.
Try CLI →Typical Use Cases
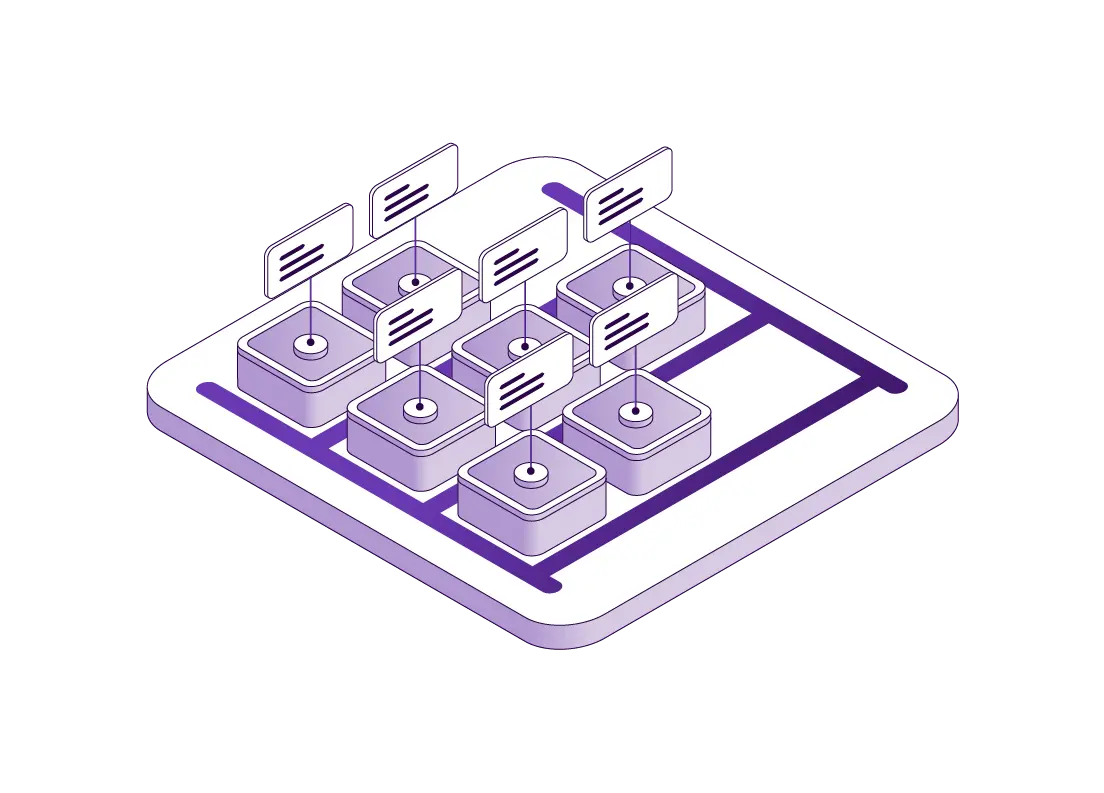
Edge Computing
Learn how to store and manage data for edge computing and AI applications.
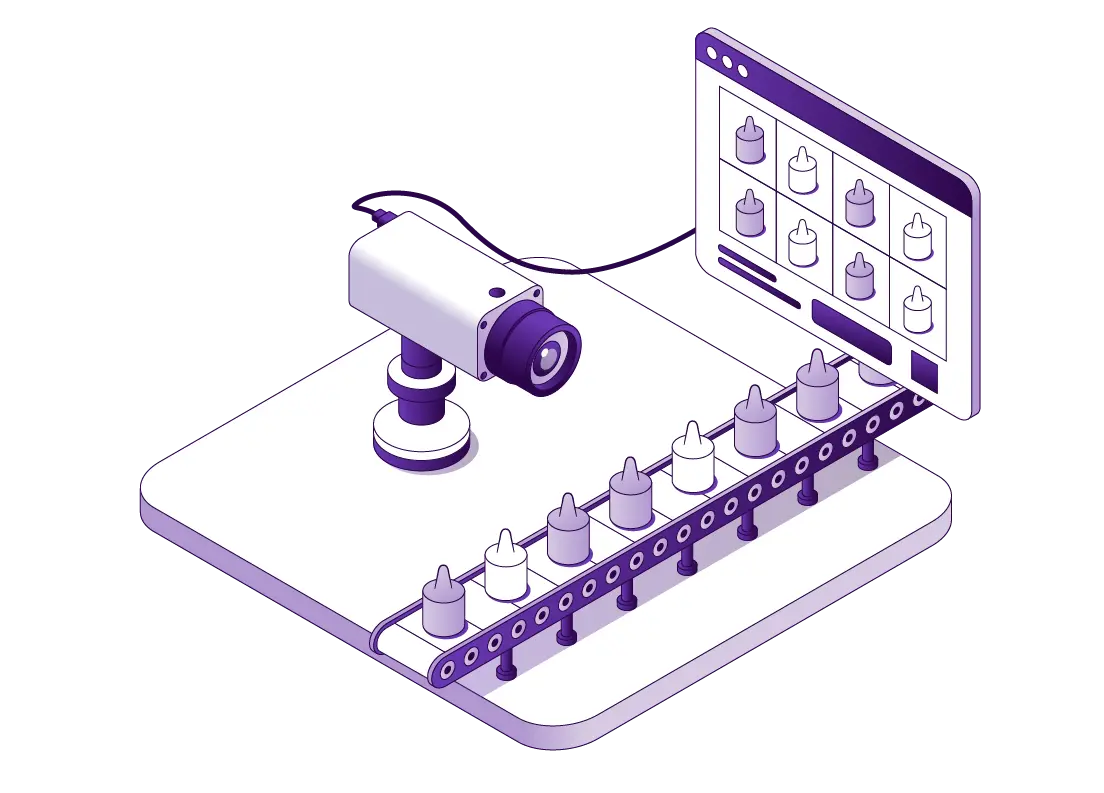
Computer Vision
Learn how to store data for computer vision applications.
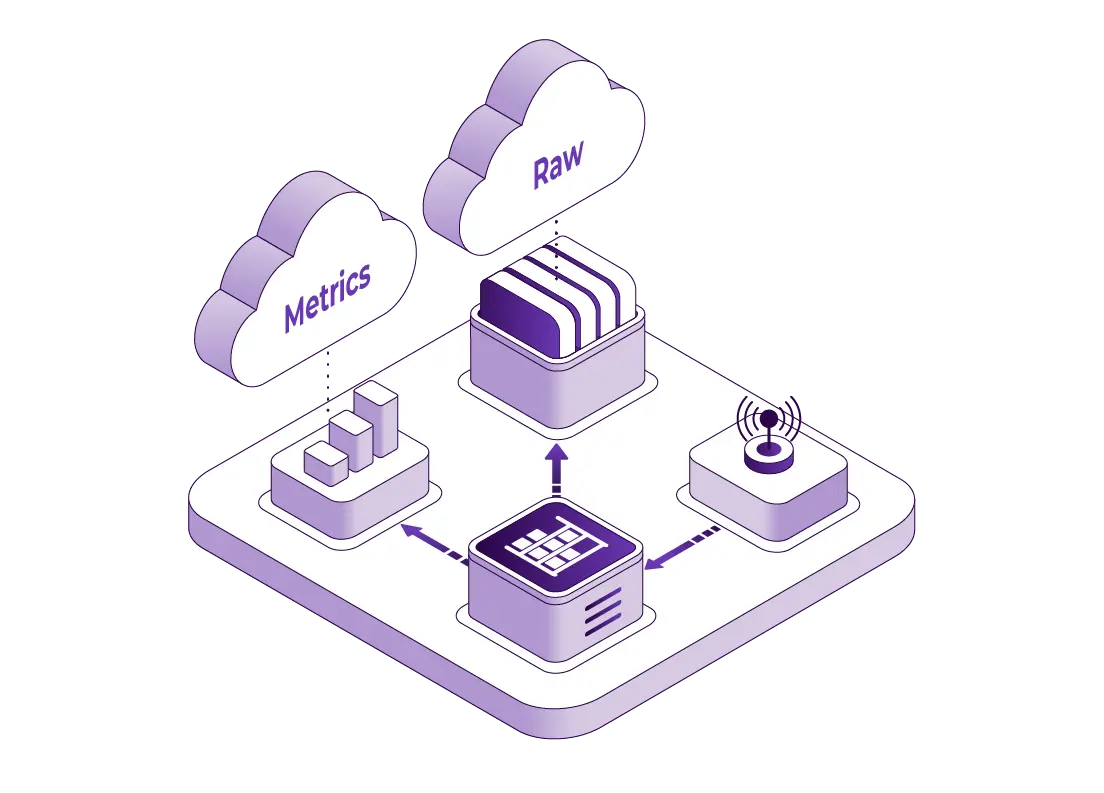
Vibration sensors
Learn how to store and manage high-frequency vibration data.
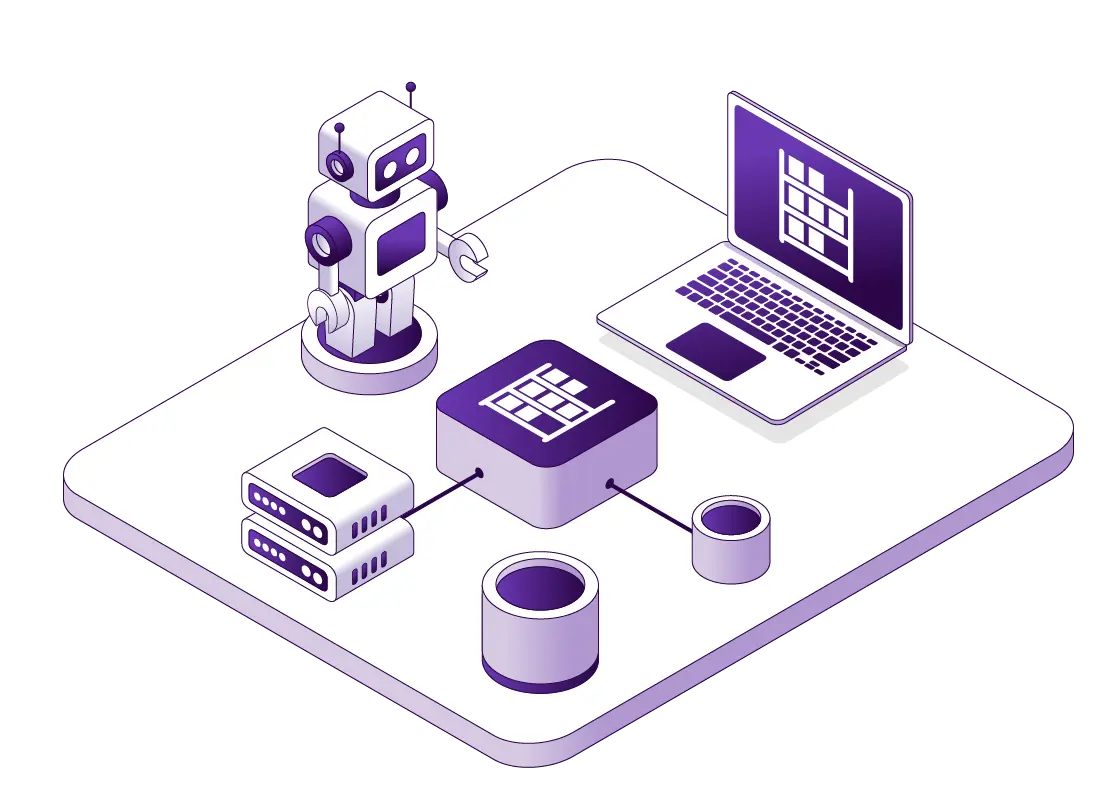
Robotics Data
Learn how to store and manage robot logs, images, and sensor data.
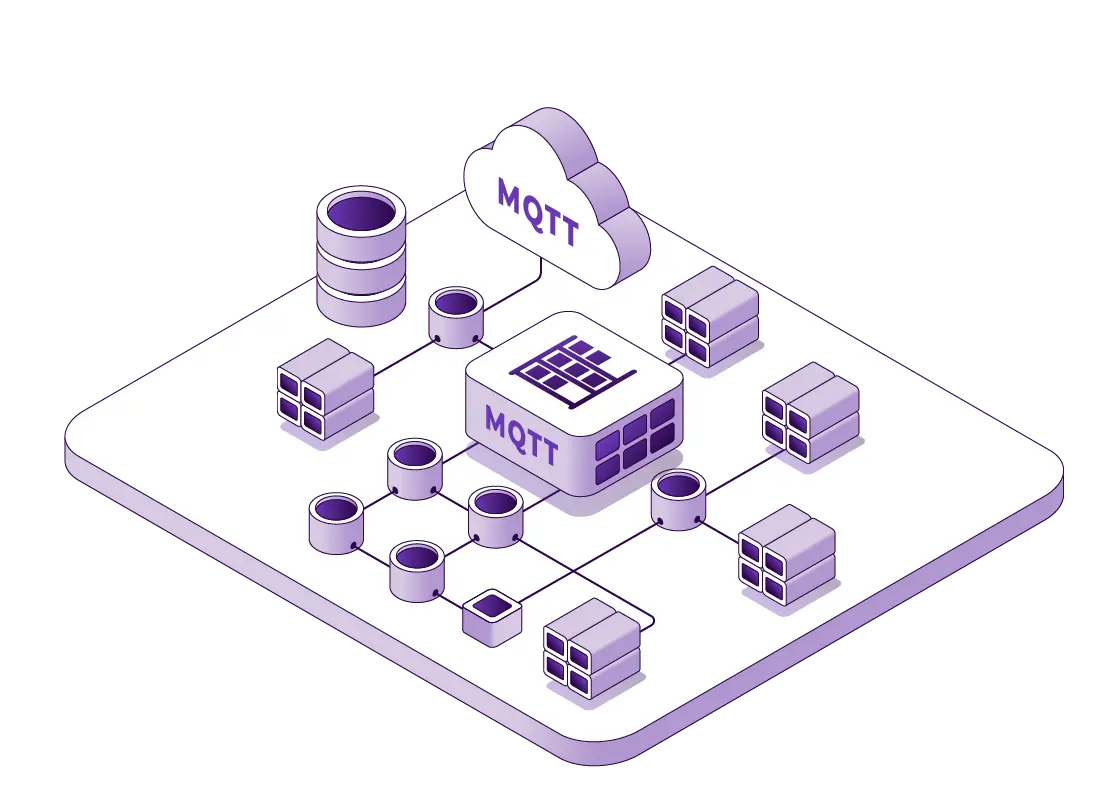
MQTT Data Storage
Learn how to store and manage MQTT data.
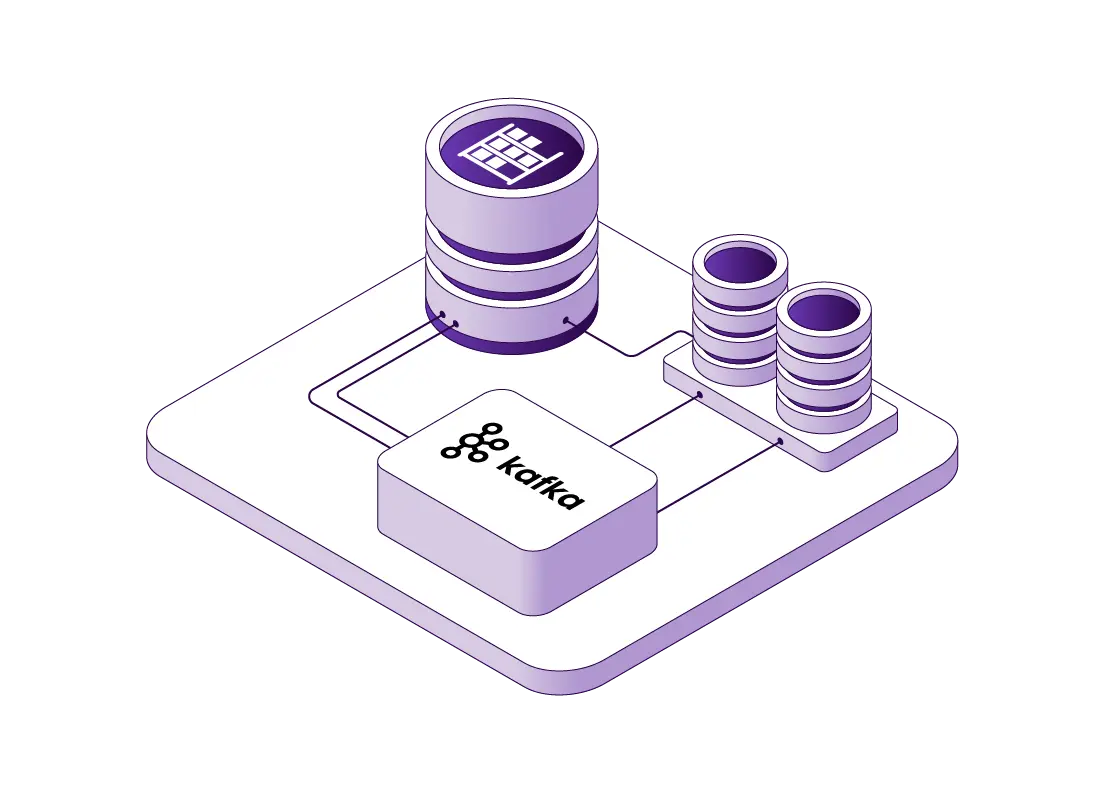
Kafka Data Sink
Learn how to implement a Kafka data sink.
Frequently Asked Questions
ReductStore is built for scalability, designed to efficiently manage large data volumes typical in edge computing and AI/ML workflows. It combines blob and time-series data functionalities, enabling the system to handle billions of time-stamped blobs. Scaling up primarily involves expanding disk capacity. The system's architecture supports low-latency access with iterator and range query capabilities, ensuring optimal performance at scale. More information about the architecture can be found in the How Does It Work section of the documentation.
Yes, ReductStore includes several customizable features for managing data, one of which is the First In, First Out (FIFO) approach. This method is specifically designed to handle data based on volume intervals, ensuring that as new entries are added, older ones are systematically removed to prevent disk space issues. Additionally, AI labels can be attached to blobs as metadata, helping in the identification and replication of key data, thus ensuring vital information is retained. These functionalities are all accessible through the ReductStore CLI, making the management of data retention and replication straightforward and effective.
Yes, ReductStore ensures compatibility with a variety of programming languages via its HTTP(S) API. Being a blob storage system, it supports the storage of all types of unstructured data in byte form, making it straightforward to incorporate into your existing setups and manage a wide range of data.
Efficient Storage & Streaming for Robotics and Industrial IoT
Learn how ReductStore helps robotics and IIoT teams manage petabytes of multimodal data-at the edge and in the cloud-with a unified solution that delivers 10x speed, 90% lower infrastructure costs, and zero data loss, even with limited bandwidth and poor connectivity.
Download White Paper (PDF)